SOLUTIONS for AI PIPELINES
The missing link: Diskover bridges the gap between AI and BI pipelines.
Diskover connects AI and BI pipelines by providing comprehensive data visibility and management, with the ultimate goal of delivering high-quality, relevant data that forms the foundation for effective analytics and machine learning.
MAKE YOUR DATA AI-READY
Transforming disorganized data into relevant, actionable datasets.
Diskover provides the ability to design unique LLMs and Multi-Modal Models tailored to your specific needs.

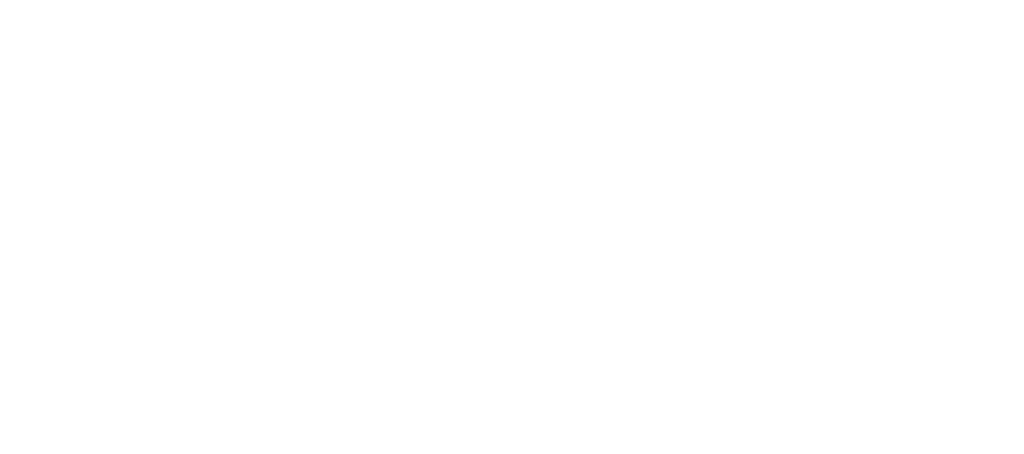
CONNECT
IMPROVE DATA QUALITY
ENHANCE LLM TRAINING
Diskover powers all AI processes.
Comprehensive data discovery and indexing.
Diskover scans and indexes vast amounts of unstructured and structured data across various storage systems. This comprehensive indexing enables organizations to locate and access relevant data swiftly, a critical step for feeding accurate and diverse datasets into AI and BI models.
Contextual metadata enrichment.
By harvesting and enriching metadata, Diskover adds context to datasets. This enriched metadata facilitates better data classification and tagging, improving the quality of data inputs for AI algorithms and enhancing the precision of BI analytics.
Data lineage and provenance tracking.
Diskover tracks data lineage, providing insights into data origins and transformations. Understanding data provenance is vital for training reliable AI models and ensuring the integrity of BI reports.
Data optimization through powerful analytics.
Diskover identifies redundant or outdated data, allowing organizations to streamline their datasets and focus on relevant, high-quality information. This ensures that AI and BI systems work with accurate, up-to-date data, enhancing the precision of analyses and predictions.
Compliance and governance.
With features that support data compliance and governance, Diskover ensures that data used in AI and BI pipelines adheres to regulatory standards. This compliance is crucial for industries with strict data handling requirements, such as healthcare and finance.
Diskover fuels AI processes with unmatched data clarity, precision, and actionable insights, turning complexities into opportunities.
Why connecting context to content is critical.
Contextual understanding.
Metadata provides additional details about the source, creation date, author, and other relevant attributes, which helps AI models interpret the raw data more accurately.
Enhanced data quality.
Identifying potential inconsistencies or errors in metadata can help improve the overall quality of the unstructured data used in AI models.
Social media analysis.
Extracting metadata like user location, sentiment, and post time from social media posts to gain deeper insights into public opinion.
Efficient data retrieval.
Metadata allows for faster and more precise retrieval of specific data points within a large dataset of unstructured information.
Unlocking hidden value.
Unstructured data often holds valuable insights that may not be readily apparent without extracting relevant metadata.
Feature engineering.
Extracted metadata can be used as additional features in machine learning models, enriching the input data and improving prediction accuracy.
Data filtering and categorization.
By extracting metadata like file type, document category, or subject, AI systems can efficiently filter and categorize unstructured data, enabling targeted analysis.
Document analysis.
Extracting metadata like document title, author, and creation date from a PDF file to categorize and prioritize documents for analysis.
Image recognition.
Using metadata like location, camera settings, and date taken to improve the accuracy of image classification.
GET STARTED WITH
DISKOVER
Ready to manage your data everywhere from anywhere?